I went to this year’s Marvell Industry Analyst Day, and the topic de jour was AI. Compared to last year (post below), this year’s industry day didn’t discuss products and strategy; it just discussed AI.
Earnings Results
Marvell reported earnings recently, and I wanted to mention that at least before I continued onwards because that is a good context-setting event for the industry day.
Revenue exceeded expectations, but the mix beneath the results was illustrative. Datacenter revenue grew 20% QoQ, cloud over 30% QoQ; while networking was strong, they guided for a 40% QoQ decline, consistent with networking OEM results.
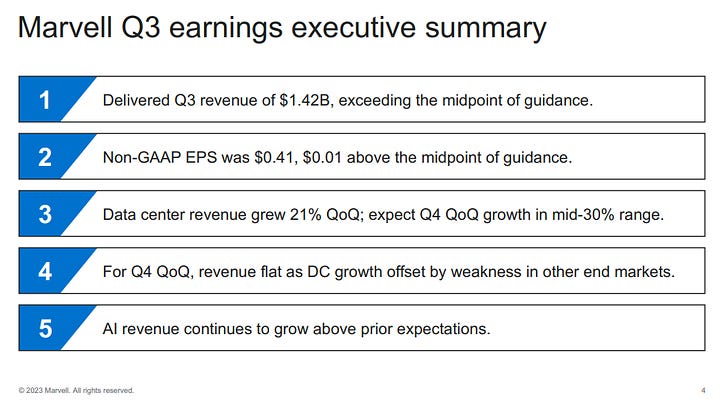
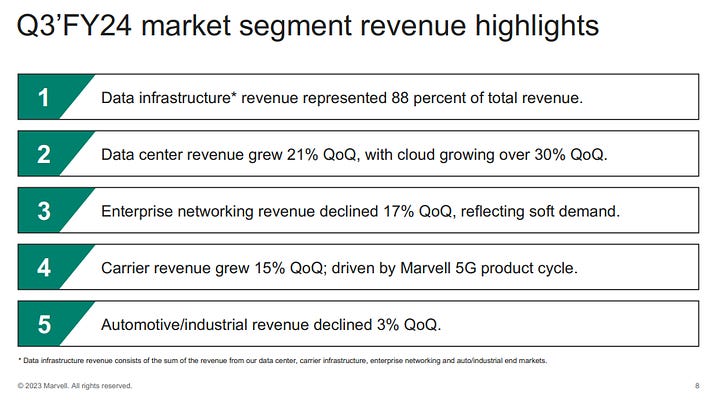
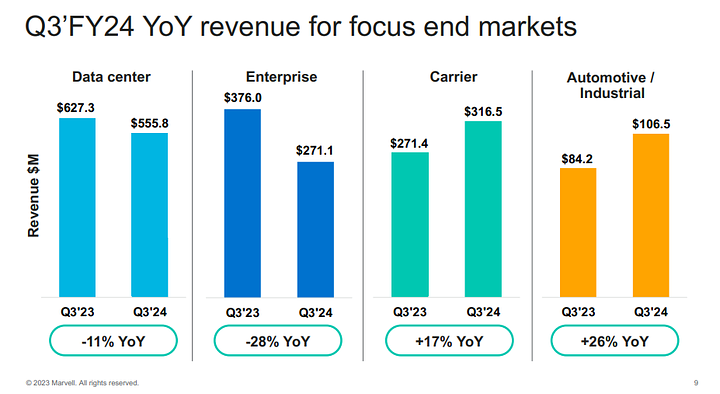
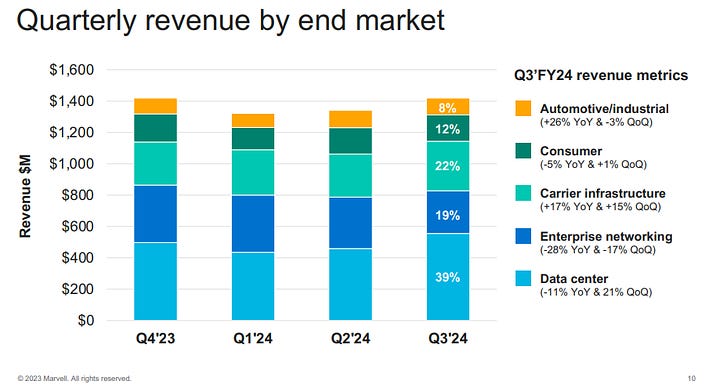
Marvell is caught in the crosswinds between cycles. The very simplistic summary is that AI is much stronger than expected, as their server attach rate continues to be strong. At the same time, carriers and networking are not as strong and are seeing the impacts of the semiconductor cycle. This is a detriment today, but if the data center turns, the carrier market will likely be strong at that point.
Now to Industry Analyst Day. The big driver is AI, and we are already starting to see its impact on Marvell’s financial guidance. They are guiding next quarter to be up 35% QoQ, and in Q4, the run-rate of AI demand will be north of 200 million. We should likely expect that run rate to remain easily for the rest of 2024, with over two billion dollars in AI revenue in 2024.
Let’s talk about how Marvell does AI, how they can benefit, and their exposure compared to the other big AI players (AMD and NVDA).
How Marvell Does AI
There are two big drivers for Marvell’s exposure to AI—networking and custom silicon. Longer-term products like CXL will move the needle, but as Marvell sits today, it’s mostly the Inphi and the custom silicon teams adding meaningful revenue. I’ll discuss each opportunity in depth.
Optics and the Bandwidth Barrier
One of the big problems in training and inferencing large models is that no model can fit on a GPU, and the way forward is to string many GPUs in parallel to train and inference large models.
GPUs are connected directly to each other in a mesh, with multiple high-speed intra-rack links to facilitate a faster data flow of large language models. AI training and inference clusters themselves have much higher speed optics than leaves in a traditional data center architecture did in the past. The pipes are fatter at the bottom compared to traditional leaf-spine architecture.
Below is a picture showing how everything an AI server touches often requires much higher bandwidth optics.
Moreover, as we scale thousands of GPUs into a cluster, Marvell will benefit from volume as each transceiver they sell has to be attached to a GPU. In the most recent conference call, they quantified it a bit better.
We've updated this number before that it had grown to greater than 1. I think that was more of a clarification we were doing. But initially, it was a little bit of how do we actually get our hands around how big this could be and people wanted to know, balance that against how many GPUs they thought were going to shift.
Now, many GPUs are connected in a mesh of Infiniband, which is not an ethernet-based switching technology, but in the future, we should expect ethernet to win the day.
A simplistic way to think about Marvell’s exposure to GPUs and AI is to take the attach rate of a DSP pluggable to a GPU and then assume their market share of the transceiver market.
I appreciated this blog post on naddod.com, which did just that: diving into the gritty details. The reference 128 H100 cluster (1024 GPUs) has 1536 800G transceivers and 935 400G transceivers. This does not include the storage transceivers, another ~500 transceivers. The attach rate is much higher than one, with about two transceivers per GPU.
Now, the cost of a DGX H100 is ~$500k per server rack on average, meaning that the vast majority of the system is approximately ~$64 million spent on DGX H100s. But if we add all the optical transceivers to the total BOM, that’s another $3.5 million in optical transceivers, of which over half will go to Marvell.
We could guess that for every ~$100 spent on a GPU, Marvell should receive something like ~$2-3 in networking in transceivers alone, compared to the total pool of $5.50 for transceivers. It would be preferable to be the GPU maker, but this is a meaningful part of the total spending in networking.
There’s a bit of a debate here that I haven’t touched: ethernet versus Infiniband. Infiband is an electrical standard (not optical), and ethernet uses optics or copper depending on the reach. Recently, Nvidia has started to make in-roads into ethernet, and with PAM4 and other modulation schemes, they have to use Marvell’s leading DSPs. Broadcom is making inroads, but Inphi within Marvell is still the largest market share holder.
Last, I need to talk about linear packaged optics or LPO. I discussed this last year during the OFC presentation and how this could be a huge detriment to Marvell. I think Marvell had a satisfying response to this problem.
I thought the Marvell team had one of the more compelling responses to the threat of linear packaged optics. That’s time to market.
The big threat is that LPO clearly should be a good solution for AI use cases. Thus, a large part of the market will be shut out to Marvell, but the problem is that LPO takes a meaningful amount of time to get to market.
For multiple switches and racks, a DSP makes sense to correct for errors between units. It makes sense that it would be first to market; according to Marvell, the first LPO solution will take ~18 months to come to market. By then, the DSP will be cheaper after ramping up scale, and the link-optimized DSP solution will be on the market.
Moreover, there is a timing issue, as 800G optics are ramping up next year in the 2024 calendar; 800 G LPO should start to show up in late 2024 or early 2025. By then, the industry will focus on 1.6T optics, which will not have an LPO solution yet. It’s just Arista’s small-scale push into LPOs, and we might never see this product in large-scale deployment.
The LPO solution might miss the market altogether. But it’s still a credible threat to Marvell’s platform. The fear is that one of the “small-scale homogeneous networks” Marvell mentions as an LPO solution is likely an AI training cluster attached to a bigger network. There is a risk that Marvell cannot get into these clusters.
I do think that the timing of the 1.6T pluggable saves them. And that Marvell’s recent 35% QoQ guide indicates AI demand is picking up. Marvell isn’t standing still either and has started to package silicon photonics for their light engine on a single chip. This will cut power costs to an industry-leading sub-ten picojoule and create a better product for future generations. Marvell is one of the leading makers of DSPs, and their Silicon Photonics shouldn’t be underestimated.
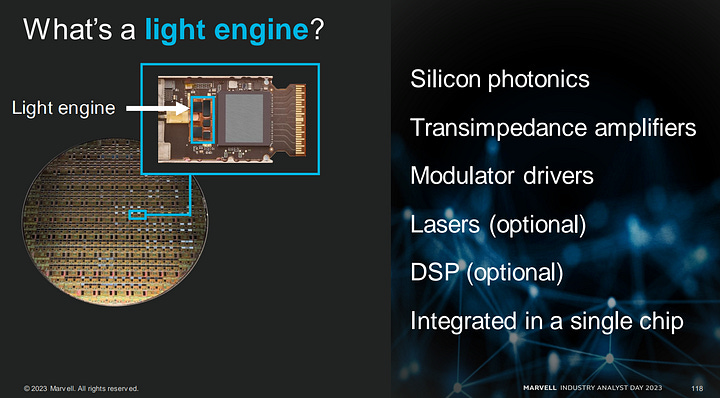
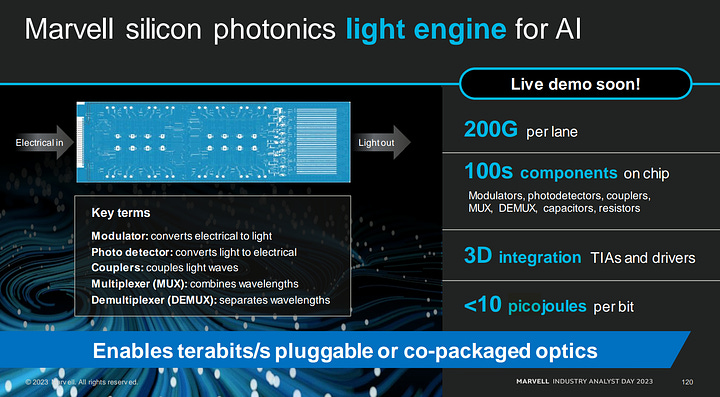
Custom Silicon
Another large and important opportunity for Marvell in AI is custom silicon. This slide showed how they support the hyperscalers in their custom silicon ambitions. Interestingly, the AI Accelerator seems to be a picture of the trainium die from Amazon.
Marvell plans to surround and support the GPU or accelerated infrastructure. They will continue to do switches (Teralynx) and interconnect their transceivers.
Interestingly, they plan to offer custom computing as an alternative solution for Nvidia’s GPUs to hyperscalers. The above picture of the trainium is a good example. Marvell will offer customization and partnership while not making the chips, supporting their ecosystem with networking or licensing IP.
This is not quite as good of a money maker as Nvidia’s flagship GPU, selling for 80% gross margins, but this also isn’t a bad business. Marvell is positioning itself as a silicon partner to the few hyperscalers worldwide, offering white-glove customization at lower margins. While this differs from the merchant silicon past, it’s where the world is going.
Marvell reasons that enough workloads can be specialized for a custom silicon solution. It might not all be an AI accelerator, and there can be other custom solutions in the future. There are only three big customers in this world of massive hyperscaler computing, so it makes sense that they would create in-house solutions. Marvell has relationships in varying degrees with each of them and expects to do more business with them in the future.
Marvell’s strategy is skating to where the world is going, not where it’s been. This market will come in at lower gross margins than their corporate average but potentially above-average operating margins. This is still a nascent opportunity that could be worth billions someday. It’s mostly the pluggable optics opportunity in AI.
Conclusion
Marvell has a credible AI revenue exposure akin to other leading AI semiconductor companies. Their analyst day highlighted how AI will change their company, and pluggable transceivers and custom silicon will be the two big vectors of growth. For more thoughts on forecasts, valuation, and the stock itself, I put my thoughts behind the paywall.